Living in the Futuristic World of AI and Healthcare
Artificial intelligence (AI) is a branch of computer science that aims to develop machines that can perform tasks that typically require human intelligence, such as understanding natural language, recognizing images, and solving problems (IBM, 2021). In recent years, AI has become increasingly prevalent in the healthcare industry due to its potential to improve positive patient outcomes, increase efficiency, and reduce costs (Arm, n.d.). AI is used in various healthcare applications, including medical imaging, drug discovery, electronic health records, and personalized medicine (Shickel et al., 2018; IBM, 2021). For instance, AI can analyze medical images to detect diseases or abnormalities that may be missed by human radiologists (Shen et al., 2020). Additionally, AI can analyze patient data to predict disease progression, identify potential risk factors, and inform treatment decisions (IBM, 2021). While there are challenges associated with its use, the benefits of AI in healthcare are significant and warrant further exploration and development. In this article, we will discuss current AI utilized in healthcare, examples of it in various healthcare settings, and the issues and implications of AI in the field of healthcare
Current AI Used in Healthcare
The use of AI in healthcare has grown rapidly in recent years, and its market size is projected to reach $45.2 billion by 2026 (Statista, 2022). The COVID-19 pandemic has further accelerated the adoption of AI in healthcare, as healthcare providers have sought to leverage AI technologies to address the pandemic's challenges (Statista, 2022). For instance, AI has been used to develop predictive models to forecast disease spread, track patient symptoms, and identify potential treatments (Wynants et al., 2020). Despite its potential benefits, the use of AI in healthcare also raises ethical and regulatory challenges. These challenges include data privacy concerns, the need for transparency and accountability in AI decision-making, and the potential for bias in AI algorithms (Obermeyer et al., 2019). Therefore, it is crucial to establish appropriate regulations and guidelines to ensure that the use of AI in healthcare is ethical and responsible (IBM, 2021). AI is a rapidly evolving field with the potential to transform healthcare by improving patient outcomes, increasing efficiency, and reducing costs.
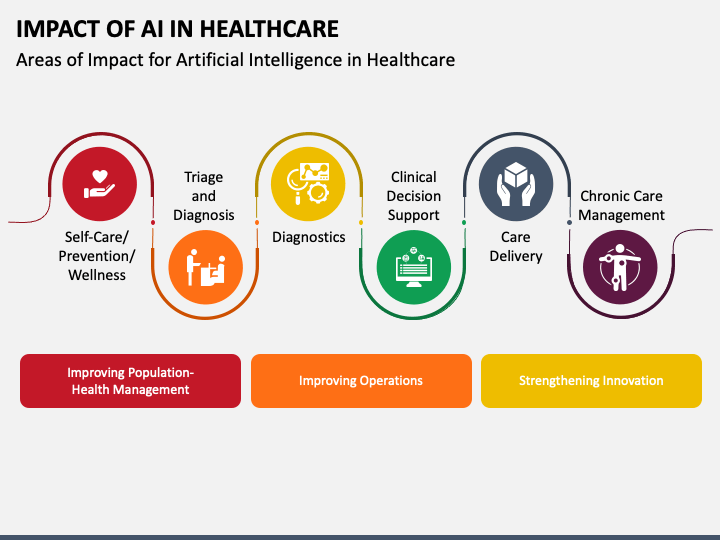
AI has become an indispensable tool in healthcare, playing a critical role in improving patient outcomes and reducing healthcare costs (Arm, n.d.). In recent years, AI has been used in a variety of healthcare applications, ranging from medical image analysis and diagnosis to drug discovery and patient monitoring. The potential benefits of AI in healthcare are vast, including more accurate diagnoses, improved treatment plans, reduced errors, and increased efficiency. This has led to an increasing number of studies exploring the use of AI in healthcare. For example, a study published in the Journal of Digital Imaging (Holli-Helenius et al., 2017) found that a deep learning algorithm could accurately identify breast cancer on mammography with a sensitivity of 85.9% and specificity of 92.5%. Similarly, a study published in the Journal of Medical Internet Research (Alvarez-Romero et al., 2022) showed that a machine learning algorithm could accurately predict readmission risk in chronic obstructive pulmonary disease (COPD) patients. Another study published in the Journal of Clinical Oncology (Lambin et al., 2021) demonstrated that an AI system could predict the response of glioblastoma (Mayo Clinic (2022) describes glioblastoma as a type of brain cancer) patients to treatment with a high degree of accuracy.
One study examined the use of AI in the detection of diabetic retinopathy, a common complication of diabetes that can lead to blindness. The study found that AI algorithms could accurately detect diabetic retinopathy in retinal images with a high level of sensitivity and specificity (Nagendran et al., 2019). Another example is the use of AI in predicting hospital readmissions for patients with heart failure. Researchers developed an AI model that could predict the likelihood of readmission based on patient data, allowing healthcare providers to intervene early and prevent readmissions (Zheng et al., 2022). In addition, AI has been utilized in the development of precision medicine approaches for cancer treatment, using data from genetic sequencing and imaging to tailor treatment plans to individual patients (Johnson et al., 2021). These examples demonstrate the potential of AI to improve healthcare outcomes and provide more personalized, effective care to patients. These studies show the potential of AI to improve healthcare outcomes and provide better patient care.
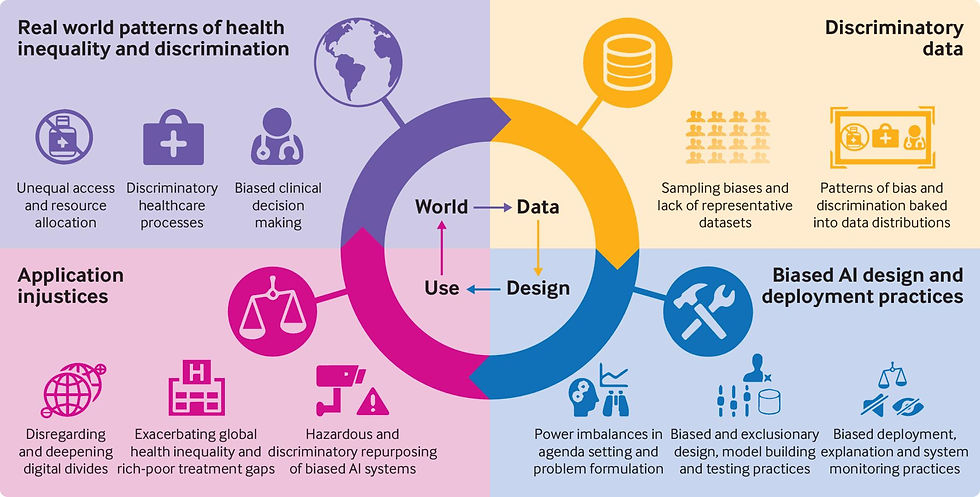
Issues and Implications of AI in Healthcare
However, as with any technology, there are challenges associated with the use of AI in healthcare, including the need for extensive and diverse datasets, ethical and legal issues, and concerns about bias and discrimination (Obermeyer et al., 2019). As the use of artificial intelligence (AI) in healthcare continues to expand, several issues and implications must be considered. While AI has the potential to improve patient outcomes and increase efficiency in healthcare settings, there are also concerns regarding the accuracy and reliability of AI algorithms, as well as issues related to patient privacy and data security (Asan & Choudhury, 2020). Another issue is the potential for AI to exacerbate existing healthcare disparities, as certain patient populations may be underrepresented in the datasets used to develop AI algorithms (Obermeyer et al., 2019). In addition, there are concerns regarding the ethical implications of using AI in healthcare, particularly concerning issues such as informed consent, data ownership, and the potential for AI to replace human decision-making (Tekkeşin, 2019). Finally, there are technical challenges related to the integration of AI systems into existing healthcare infrastructure, such as compatibility with electronic health records and ensuring that AI algorithms are validated and clinically relevant (Topol, 2019). These issues and implications must be carefully considered to ensure that AI is used responsibly and effectively in healthcare settings.
Conclusion
In conclusion, the use of artificial intelligence (AI) in healthcare has become increasingly prevalent in recent years, with the potential to transform healthcare by improving patient outcomes, increasing efficiency, and reducing costs (Arm, n.d.; IBM, 2021). While the benefits of AI are significant, there are also challenges associated with its use. Ethical and regulatory challenges such as data privacy concerns, transparency and accountability in AI decision-making, and potential bias in AI algorithms need to be addressed through appropriate regulations and guidelines to ensure that the use of AI in healthcare is ethical and responsible (Asan & Choudhury, 2020; Obermeyer et al., 2019). There are also examples of AI being used to accurately identify breast cancer, predict readmission risk in patients with chronic obstructive pulmonary disease, and predict the response of glioblastoma patients to treatment with a high degree of accuracy (Alvarez-Romero et al., 2022; Holli-Helenius et al., 2017; Lambin et al., 2021). Despite the potential benefits, the need for extensive and diverse datasets, ethical and legal issues, and concerns about bias and discrimination, as well as technical challenges related to the integration of AI systems into existing healthcare infrastructure, are some of the challenges that need to be addressed (Obermeyer et al., 2019; Topol, 2019). However, with appropriate regulations and guidelines, the use of AI in healthcare has the potential to improve healthcare outcomes and provide more personalized, effective care to patients (Davenport & Kalakota, 2019). Therefore, researchers, healthcare professionals, and policymakers need to work together to ensure the responsible and ethical use of AI in healthcare.
Bibliographical References
Alvarez-Romero, C., Martinez-Garcia, A., Ternero Vega, J., Díaz-Jimènez, P., Jimènez-Juan, C., Nieto-Martín, M. D., Román Villarán, E., Kovacevic, T., Bokan, D., Hromis, S., Djekic Malbasa, J., Beslać, S., Zaric, B., Gencturk, M., Sinaci, A. A., Ollero Baturone, M., & Parra Calderón, C. L. (2022). Predicting 30-Day Readmission Risk for Patients With Chronic Obstructive Pulmonary Disease Through a Federated Machine Learning Architecture on Findable, Accessible, Interoperable, and Reusable (FAIR) Data: Development and Validation Study. JMIR medical informatics, 10(6), e35307. https://doi.org/10.2196/35307
Arm. (n.d.). AI in healthcare. Arm Glossary. Retrieved March 28, 2022, from https://www.arm.com/glossary/ai-in-healthcare
Choudhury, A., & Asan, O. (2020). Role of Artificial Intelligence in Patient Safety Outcomes: Systematic Literature Review. JMIR medical informatics, 8(7), e18599. https://doi.org/10.2196/18599
Davenport, T., & Kalakota, R. (2019). The potential for artificial intelligence in healthcare. Future healthcare journal, 6(2), 94–98. https://doi.org/10.7861/futurehosp.6-2-94
Holli-Helenius, K., Salminen, A., Rinta-Kiikka, I., Koskivuo, I., Brück, N., Boström, P., & Parkkola, R. (2017). MRI texture analysis in differentiating luminal A and luminal B breast cancer molecular subtypes - a feasibility study. BMC medical imaging, 17(1), 69. https://doi.org/10.1186/s12880-017-0239-z
IBM. (2021). Artificial intelligence. IBM. Retrieved March 28, 2022, from https://www.ibm.com/topics/artificial-intelligence
IBM. (2021). Artificial intelligence in healthcare. IBM. Retrieved March 28, 2022, from https://www.ibm.com/topics/artificial-intelligence-healthcare
Johnson, K. B., Wei, W. Q., Weeraratne, D., Frisse, M. E., Misulis, K., Rhee, K., Zhao, J., & Snowdon, J. L. (2021). Precision Medicine, AI, and the Future of Personalized Health Care. Clinical and translational science, 14(1), 86–93. https://doi.org/10.1111/cts.12884
Lambin, P., Leijenaar, R. T. H., Deist, T. M., Peerlings, J., De Jong, E. E. C., Van Timmeren, J., Sanduleanu, S., & Oberije, C. (2021). Radiomics and AI for Response Prediction in Glioblastoma Patients: A Multi-Institutional Study. Journal of Clinical Oncology, 39(15_suppl), 2016–2016. https://doi.org/10.1200/JCO.2021.39.15_suppl.2016
Mayo Clinic. (2022). Glioblastoma. Mayo Clinic. Retrieved March 29, 2022, from https://www.mayoclinic.org/diseases-conditions/glioblastoma/symptoms-causes/syc-20350234
Nagendran, M., Chen, Y., Lovejoy, C. A., Gordon, A. C., Komorowski, M., Harvey, H., Topol, E. J., Ioannidis, J. P. A., Collins, G. S., & Maruthappu, M. (2020). Artificial intelligence versus clinicians: systematic review of design, reporting standards, and claims of deep learning studies. BMJ (Clinical research ed.), 368, m689. https://doi.org/10.1136/bmj.m689
Obermeyer, Z., Powers, B., Vogeli, C., & Mullainathan, S. (2019). Dissecting racial bias in an algorithm used to manage the health of populations. Science, 366(6464), 447–453. https://doi.org/10.1126/science.aax2342
Shen, D., Wu, G., & Suk, H. I. (2017). Deep Learning in Medical Image Analysis. Annual review of biomedical engineering, 19, 221–248. https://doi.org/10.1146/annurev-bioeng-071516-044442
Shickel, B., Tighe, P. J., Bihorac, A., & Rashidi, P. (2018). Deep EHR: A survey of recent advances in deep learning techniques for electronic health record (EHR) analysis. IEEE Journal of Biomedical and Health Informatics, 22(5), 1589–1604. https://doi.org/10.1109/JBHI.2017.2767063
Statista. (2022). AI in healthcare market size worldwide from 2018 to 2026 (in billion U.S. dollars). Statista. Retrieved March 28, 2022, from https://www.statista.com/statistics/1334826/ai-in-healthcare-market-size-worldwide/
Statista. (2022). AI in healthcare. Statista Dossier. Retrieved March 28, 2022, from https://www.statista.com/topics/10011/ai-in-healthcare/#dossier-chapter3
Tekkeşin A. İ. (2019). Artificial Intelligence in Healthcare: Past, Present and Future. Anatolian journal of cardiology, 22(Suppl 2), 8–9. https://doi.org/10.14744/AnatolJCardiol.2019.28661
Topol, E. J. (2019). High-performance medicine: the convergence of human and artificial intelligence. Nature Medicine, 25(1), 44–56. https://doi.org/10.1038/s41591-018-0300-7
Wynants, L., Van Calster, B., Collins, G. S., Riley, R. D., Heinze, G., Schuit, E., Bonten, M. M. J., Dahly, D. L., Damen, J. A. A. G., Debray, T. P. A., de Jong, V. M. T., De Vos, M., Dhiman, P., Haller, M. C., Harhay, M. O., Henckaerts, L., Heus, P., Kammer, M., ... Van den Bruel, A. (2020). Prediction models for diagnosis and prognosis of covid-19: Systematic review and critical appraisal. BMJ, 369, m1328. https://doi.org/10.1136/bmj.m1328
Zheng, L., Smith, N. J., Teng, B. Q., Szabo, A., & Joyce, D. L. (2022). Predictive Model for Heart Failure Readmission Using Nationwide Readmissions Database. Mayo Clinic proceedings. Innovations, quality & outcomes, 6(3), 228–238. https://doi.org/10.1016/j.mayocpiqo.2022.04.002
Visual Sources
Comments